Test Drive: text to SQL
Published on .
Today I’m test driving Qwen2.5 for a text to sql task. I’m not going to use anything special. I’ve heard great things about the use of <xml>
tags so I’m starting there, like this:
<schema>{schema}</schema>
<question>{question}</question>
<sql>
From there, I just used a small, quantized Qwen: mlx-community/Qwen2.5-Coder-1.5B-Instruct-8bit
I added a pretty printer, which is of course independent of the LLM.
I’m impressed with the output, which includes join
s and more, as you can see in the screenshot below.
To use it:
- Format your schema as
CREATE TABLE AS
statements in actas
variable. - call
prompt.format(question="your question here", schema=ctas)
- Pass that to the model
Here’s some example output (which I accidentally lowercased)
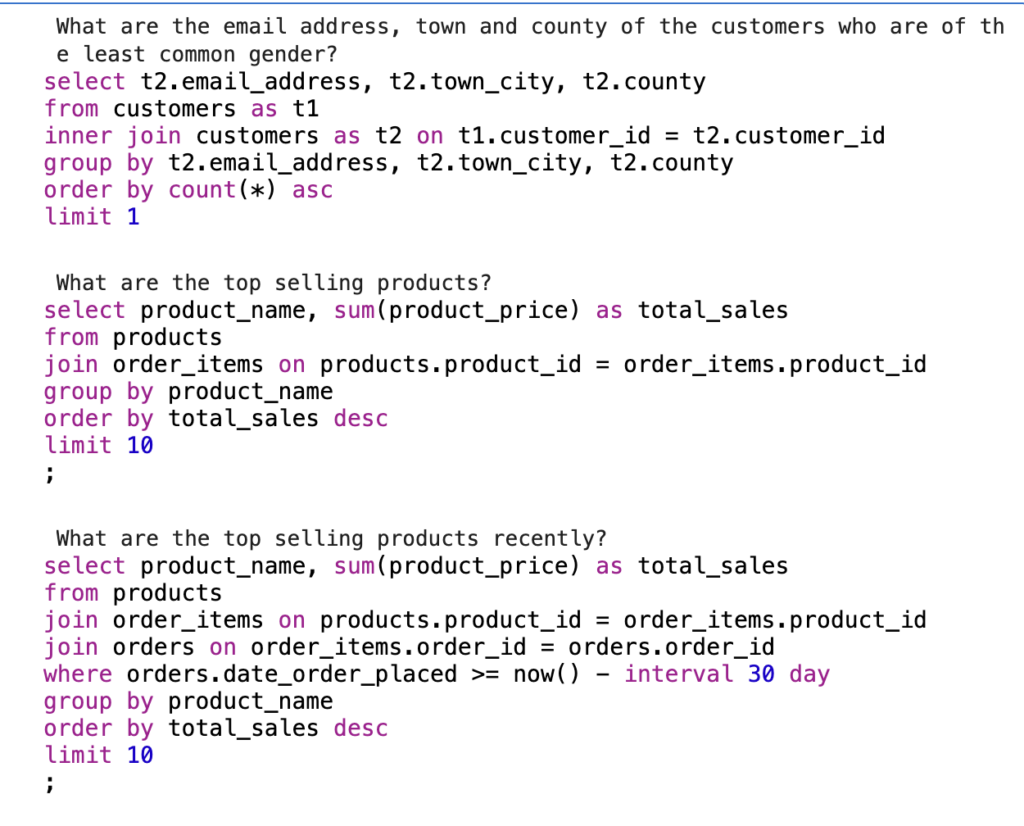
from sqlfmt.api import Mode, format_string
from rich.console import Console
from rich.syntax import Syntax
def pretty(q):
console = Console()
syntax = Syntax(format_string(q, Mode()), "sql", theme="xcode")
console.print(syntax, style="on white")
questions = [
"What are the email address, town and county of the customers who are of the least common gender?",
"What are the top selling products?",
"What are the top selling products recently?",
]
for q in questions:
print(q)
messages = [{"role": "user", "content": text.format(question=q, schema=ctas)}]
prompt = tokenizer.apply_chat_template(
messages, tokenize=False, add_generation_prompt=True
)
pretty(generate(model, tokenizer, prompt=prompt, verbose=False, max_tokens=2000))
"""Text to SQL"""
from mlx_lm import load, generate
model, tokenizer = load("mlx-community/Qwen2.5-Coder-1.5B-Instruct-8bit")
ctas = """CREATE TABLE products (
product_id number,
parent_product_id number,
product_name text,
product_price number,
product_color text,
product_size text,
product_description text);
CREATE TABLE customers (
customer_id number,
gender_code text,
customer_first_name text,
customer_middle_initial text,
customer_last_name text,
email_address text,
login_name text,
login_password text,
phone_number text,
address_line_1 text,
town_city text,
county text,
country text);
CREATE TABLE customer_payment_methods (
customer_id number,
payment_method_code text);
CREATE TABLE invoices (
invoice_number number,
invoice_status_code text,
invoice_date time);
CREATE TABLE orders (
order_id number,
customer_id number,
order_status_code text,
date_order_placed time);
CREATE TABLE order_items (
order_item_id number,
product_id number,
order_id number,
order_item_status_code text);
CREATE TABLE shipments (
shipment_id number,
order_id number,
invoice_number number,
shipment_tracking_number text,
shipment_date time);
CREATE TABLE shipment_items (
shipment_id number,
order_item_id number);
"""
text = """Generate SQL to answer the question given the schema.
Do not explain, ONLY OUTPUT SQL !!!
<schema>{schema}</schema>
<question>{question}</question>
<sql>"""
q = "What are the email address, town and county of the customers who are of the least common gender?"
q = "What are the top selling products?"
messages = [{"role": "user", "content": text.format(question=q, schema=ctas)}]
prompt = tokenizer.apply_chat_template(
messages, tokenize=False, add_generation_prompt=True
)
print(generate(model, tokenizer, prompt=prompt, verbose=False, max_tokens=2000))